Three weeks ago I published a translation I made of a scene in Alex Schulman‘s Överlevarna (The Survivors). To do so I made use of machine translation software available on-line. Two weeks ago my post was an overview of on-line translation software. From the beginning my plan was to share the translation and then discuss using on-line translation software to make it. I lost momentum when the second article took so many more words than I’d anticipated. Then came last week’s unplanned wisdom tooth digression/extraction. This week, let’s see if I can get back on track!
Machine translation and literary texts
Translation software is not intended for literary texts. Or if it is intended for literary texts, it is still far from an ideal tool. In this piece I’ll tell you why, with concrete examples from the passage about the children’s swimming race from Alex Schulman’s novel.
To the best of my knowledge, all machine translation software depends principally on three things. A large database of pre-translated texts, a rapid capacity to search the database, and an algorithm to pick out the most likely translation of several options for any given input.
The software does not create a translation. It’s not capable of creativity. Instead it breaks the input text down into grammatical groups and then searches in its database for existing translations of sentences, phrases or words. Then it uses algorithms to assign a probability to the translations it finds. “This is the most likely translation,” it says. “Or maybe this, or this.”
The human asking for the translation is supposed to pick and choose among the options. Most don’t. The reason they’re asking for the translation in the first place is because they don’t know the language they’re translating into. They are not in a position to pick the right word. And mostly it doesn’t matter.
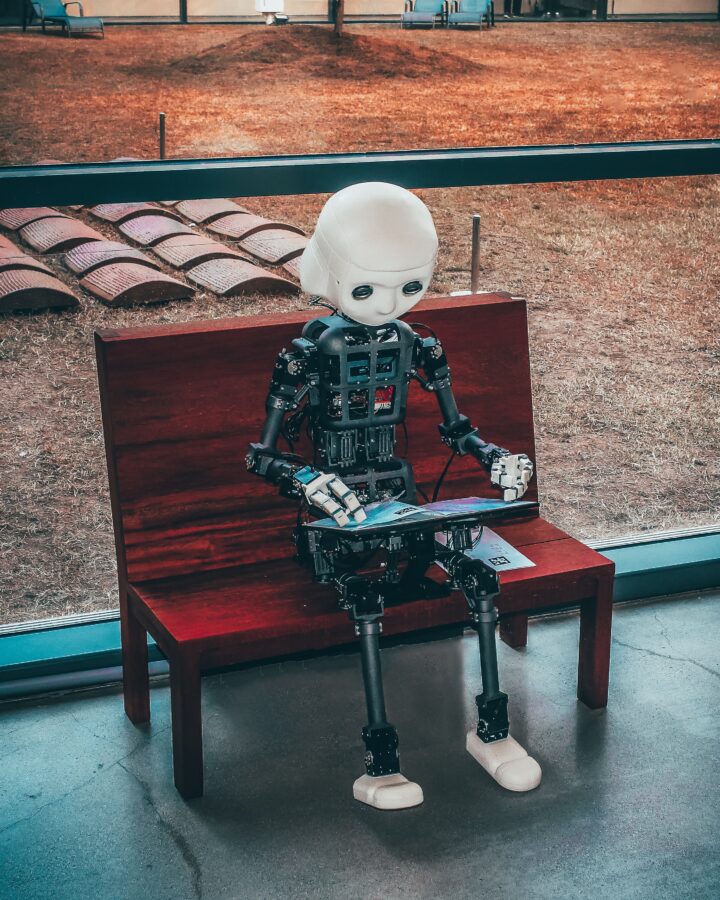
Using mechanical translation
What we use mechanical translation for is to get the gist of a text in another language than our own. To find out what a friend has written, perhaps on social media. To get an idea of the information in an official document, an advert or a newspaper article. Or to offer others a version of a text that we’ve produced in our own language.
It’s this last one that’s the most tricky. If you translate something into your own language, you can usually spot when the mechanical translation has failed. You can use your own knowledge of the language to interpret the words the software has given you. But you are unlikely to be able to do the same when the software has turned your writing into another language. And this is particularly a problem with literary texts.
Let’s look at some of the difficulties the mechanical translation software had with the Swedish text I translated three weeks ago.
Everyday expressions
Jag ska ta tid, says Benjamin’s father. He’s going to be the timekeeper for the race. I translate this as: I’ll keep time. Google translates it as I’ll take time. (This is the literal, word-for-word translation.) DeepL, weirdly, translates it as I’ll make time. The problem here is that the expression has a precise meaning that is not the same as the literal sense of the separate words. It’s possible the expression is to be found in both Google’s and DeepL’s databases, but not with a high probability. Neither programme takes a wide enough perspective of the text to see that ta tid relates to timekeeping.
When the boys are getting ready to run down to the lake, the father says: På edra platser. This is an even more fixed expression and there is an equivalent fixed expression in English: On your marks. But again the software fails to identify it.
Curse words
Curse words and taboo words are present in every language. They are often among the words we are most keen (as children) to learn in a foreign language simply because they are forbidden. (OK, I speak for myself here, but maybe for you too!) You may not believe me, but curse words are very delicate words when it comes to translation. The same person may use the same swear words in very different ways, and for very different purposes. Swear words may also indicate things that are not obvious, like irritation or friendliness, social cohesion or social distance.
The boys’ father mutters to himself when he can’t set his watch to time the race. His thumbs are too big for the little buttons he must press. Vafan he says, in irritation. A literal translation would be something like what the devil. Google doesn’t even attempt a translation, while DeepL tries what the hell. I go with a muttered fuck. Looking at it again, though, I wonder if that’s not too strong. He’s not talking to his boys, but of course he’s aware they are listening. Perhaps what the hell would be a better translation. On the other hand, he’s a drunk with a short temper.
The boys mess about, pushing one another before the race starts, and both parents react. The father tells them off and their mother threatens to abandon the race: Då skiter vi i det. Google: Then we shit in it; DeepL: Then screw it. Again, Google has a word-for-word literal translation, DeepL’s is more emotive. Both translations seem far from the actual sense. The mother is not being potty-mouthed, even though she is also drunk. Swedish skita i is a phrasal verb meaning something like not give a damn. My translation went for the sense. Following on from the father’s reprimand – None of that! – the mother says Or we’ll just forget it.
Spoken language
As I wrote above, mechanical translation software draws on a database of written material. This is especially true of Google Translate which started out using large databases of legal and factual texts that had already been professionally translated. For example the body of texts produced within the EU, translating essential information into all the languages of EU member states. (DeepL is qualitatively different, which may reflect a more varied range of texts in its database.) Still, you’d expect the software to stumble over spoken forms. And indeed it does, though DeepL less than Google.
As the boys swim out to the buoy, they become more and more tired and cold. They slow down and draw together. The eldest brother, Nils, swimming ahead, looks like he’s getting into trouble. Benjamin, the middle brother, swims closer to him and asks Hur är det? Google renders this literally: How is it? DeepL translates it as: What’s up? I went with the DeepL translation, but I look at it now and wonder if How are you doing? might not be better. Benjamin is worried.
Nils says: Jag vet inte om jag klarar det här. Google translates this in stilted language as I do not know if I can handle this. Literally correct, but somehow missing the point. DeepL suggests: I don’t know if I can do this. Better. Maybe I could have written: I don’t know if I can manage this. But I went with DeepL’s translation.
Unusual vocabulary and multiple senses
Another area where translation software fails is with unusual words and words that have multiple senses.
Remembering fishing expeditions on the lake with their father, Benjamin describes how pappa plockade fiskar ur nätet och kastede i durken. Durken? I’m lost and I can’t find a likely translation – I just know Google and DeepL are both wrong. Google gives me: Dad picked fish out of the net and threw in the floor. The preposition is wrong, there’s a noun or pronoun missing, and floor? How does it get that? But DeepL is just as obscure, if grammatically more accurate: father picked fish out of the net and threw them in the trough.
Logically, I thought, durken must mean the bucket. What else would you toss fish into? So I wrote that, till I thought to ask Mrs SC. Durken is the bottom of the boat, she said, the boards you put your feet on. And she gave me a look that said “obviously”. So I went looking and found these boards are called bottom-boards in English. Which gave me: father picked fish out of the net and tossed them on the bottom-boards.
In the same section, remembering the fish on the bottom-boards, Benjamin thinks of how fiskarna slog. That looks like the fish fought. But Google interpreted it as the fishermen struck! Fiskarna can be read as the fishes or as the fishers, and slog can be fought or struck. DeepL gave me the fish struck. Now I have visions of fishes (or fisherfolk) on strike, parading around with protest signs. I went with the fish thrashed suddenly, which I thought was truer to the sense of the passage.
Metaphor and simile
This is where mechanical translation often breaks down completely. Not that there was so much imagery in this extract, but there was enough to illustrate the point.
As the boys start swimming out to the buoy, the lake’s surface is spegelblank (den spegelblanka sjön). The sense is that the surface is still and clear like the surface of a mirror. This is no neologism, it may even be a cliché. (I’ve certainly come across it before.) But there is, apparently, no established translation. Google translates it as the mirror-shiny lake – mirror shiny being a literal translation of spegel blanka. But this doesn’t work in English. DeepL offers the mirror-white lake. This is because of a second meaning of blanka, but it is even less accurate than the Google translation. I went for the mirror-clear lake. But searching for phrases online now I find mirror-bright seems to have been used in English before. Probably I should have opted for that.
On the return, Benjamin sees the family’s house som en röd legobit. An appropriate comparison for a nine-year old to make. Google has him see the house as a red Lego piece. DeepL puts i better: The house like a red Lego piece. But I go for: The house like a piece of red lego. (And deliberately choose a lowercase L for Lego – both Google and DeepL are too respectful of trademarks!)
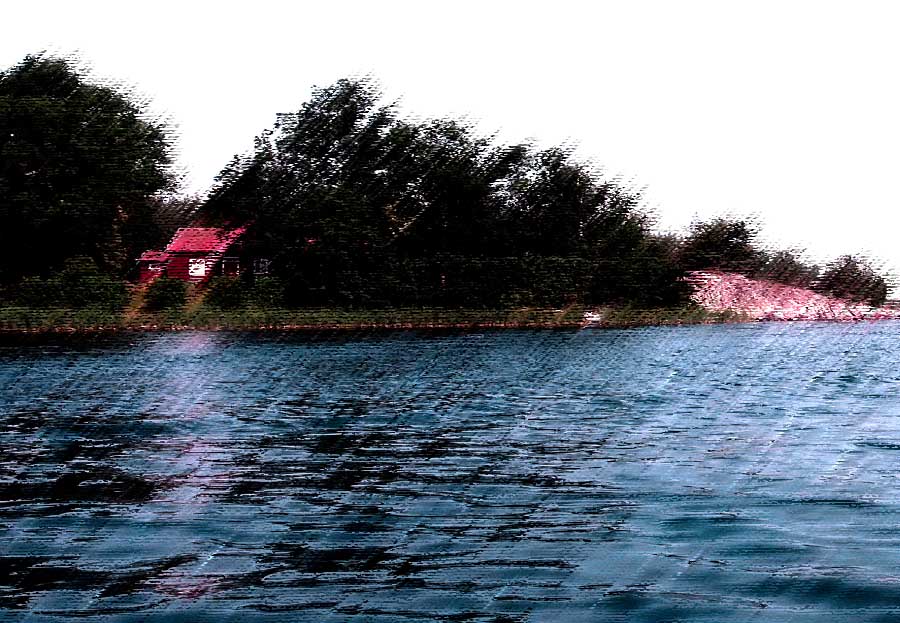
The final sentence of the extract carries all the weight of the boys’ exhaustion, confusion and disappointment: Tre oroliga andhämtningar ute i tystnaden. This is poetry, and it is way beyond machine translation. Google offers: Three anxious breaths out in the silence. And DeepL: Three anxious gasps out in the silence. Neither capture the anthropomorphism of the original. I’m not sure mine does either, but this was how I tried: Three uneasy gaspings alone in the silence.
Choosing translators
Where does all this get us? Here, I think.
If you want a literary text translated into a foreign language, it is better by far to employ a qualified human being to make the translation. If you can’t persuade anyone to help, not for love or money, if their eyes glaze over when you raise the subject. Stop! Consider if your text really needs to be translated. Consider if your text is as good as you can make it in your own language. (It’s worth remembering the GIGO rule – Garbage In is Garbage Out.)
But if you have to have the text translated, (and if you are translating to one of the languages DeepL supports), then choose DeepL. It’s not perfect and will provoke both confusion and laughter in your readers, but it will make a text closer to your meaning that Google (or Bing).
And that is all I want to write about translating for a good while!
Picture credits
The little robot reading on a bench is by Andrea De Santis on Unsplash
The red cottage by the lake in the evening is based on a photo in my own archives.
Great topic, John! I do write and translate back and forth between English and German and a friend recommended DeepL to me. I tested it out and it was not bad – as long as you are fairly competent in both languages, at least. Which I happen to be. So I took up DeepL’s offer of a month’s free trial and tossed my backlog of stories through it – short ones and even a novel. I must say, the speed with which they tossed the stories back at me was dizzying! If it’s a story I wrote in German, it’s a cinch to bring it up to speed in English, but having the DeepL version expedites the process for me. A story of mine written in English and tossed into German takes me a little longer. They (meaning DeepL) translate idiomatic expressions literally and sometimes I’m not quite sure if it’s legitimate to say it like that in German. And it’s often not. That’s what German native speakers are good for. My son is one in fact. Great to have a home-grown expert at my disposal.
Idiom is an eternal problem for machine translation. If an idiom is so well established the machine knows it, it’s probably on its way out in human daily use. Everything else, yes, the machines interpret literally. I don’t think machine translation is ever going to seriously challenge human translators, but there are plenty of people with no ear for language and no money to spend on translators who will happily couple their websites to Google and live with the results. At least that gives the rest of us something to laugh at.
🙂